About Us
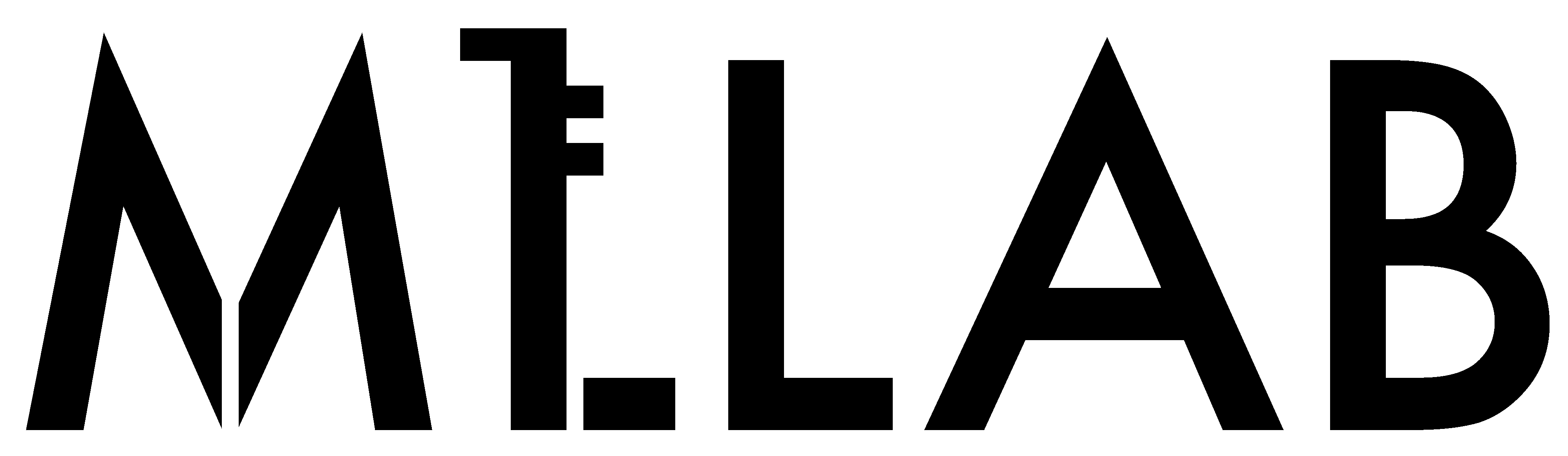
The Machine Learning Lab at Seoul National University focuses on developing principled optimization algorithms to address fundamental challenges in machine learning. These challenges often involve combinatorial, high-dimensional, or computationally intensive objectives, arising in areas such as neural network compression, metric learning, reinforcement learning, and adversarial robustness. By leveraging techniques such as combinatorial optimization, algebraic, and probabilistic methods, the lab designs efficient and scalable solutions for these complex tasks.
A unifying theme of the lab's work is the development of algorithms that are both theoretically grounded and practically impactful. This includes exploiting problem structures when possible, while ensuring robustness and adaptability in less predictable environments, such as those encountered in reinforcement learning. Central questions drive the lab's research: How can optimization frameworks scale effectively with modern computational demands? What strategies enable robust and adaptive learning across diverse applications? By addressing these questions, the lab aims to advance machine learning with methodologies that are rigorous, efficient, and widely applicable.