Puzzle Mix: Exploiting Saliency and Local Statistics for Optimal Mixup
August 27, 2020
Article by Jang-Hyun Kim and Wonho Choo
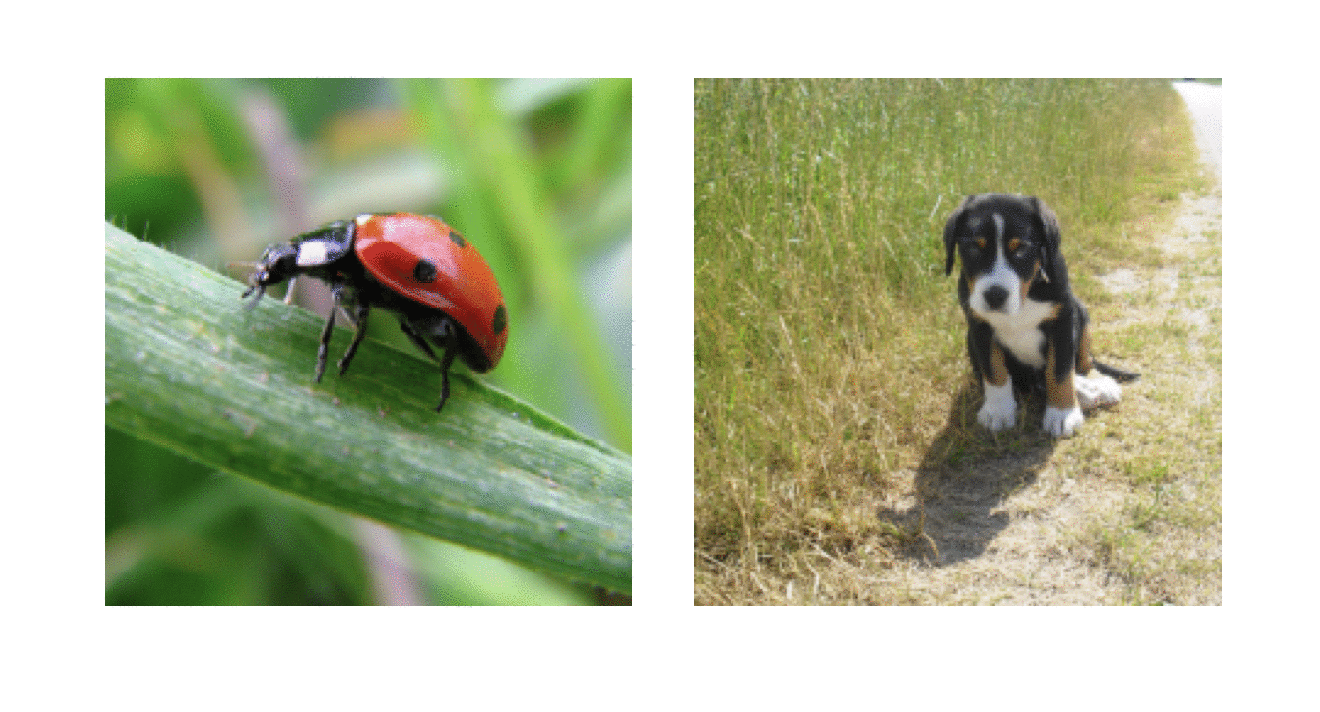
Deep neural networks become the bedrock of modern AI tasks such as object recognition, speech, natural language processing, and reinforcement learning. However, these models are known to memorize the training data and make overconfident predictions often resulting in degraded generalization performance on test examples [Zhang2016]. Data augmentation approaches aim to alleviate some of these issues by improving the model generalization performance [Bishop2006].
Recently, a line of new data augmentation techniques called mixup has been proposed [Zhang2018]. These methods mainly focus on creating previously unseen virtual mixup examples by interpolating a pair of given data. In this article, we will cover these methods with our paper “Puzzle Mix: Exploiting Saliency and Local Statistics for Optimal mixup”.
Read more